Machine learning for emergency response
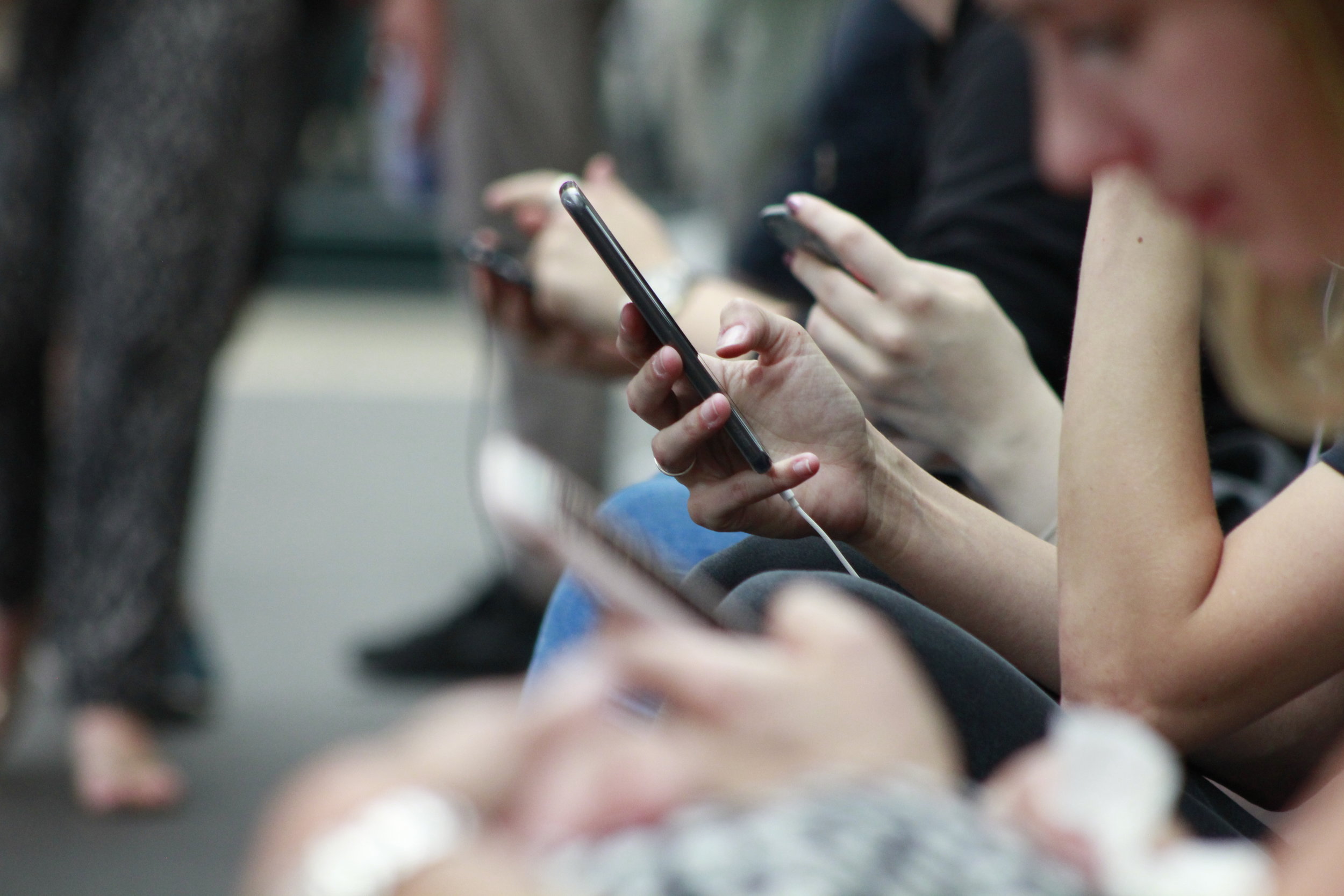
About this project
Hurricane Harvey was a devastating Category 4 hurricane that made landfall on Texas and Louisiana in August 2017, causing catastrophic flooding and more than 100 deaths. During the hurricane, 911 hotlines in Houston were overwhelmed, and in the aftermath it was clear there was a need to inovate on current emergency response systems.
Funded by an NSF grant, our team of researchers built a system to recognize those in need of rescuing based on private social media images and machine learning image classification models. This idea does not replace emergency response systems, but provides an innovative way to utilize communication on private channels.
My role
I managed the processes between our fieldworkers and computational team. As one of three model trainers, I focussed on data processing, evaluation, and reporting. I also authored a paper and presented it at the IEEE ICMLA 2018 conference.
The outcome of our project was an image classifier, trained on signal data, that categorizes private and public social media imagery between three categories: rescuer, volunteer rescuer, & rescuee.
More about the modeling
Few crisis informatics studies have trained on highly curated, non-public social media data gathered from the field. The graphic below summarizes our literature review findings and illustrates why “signal” social media data (private messages) is the focus of our project.
The model developed in this project has potential application as an alternative emergency response system, highlighting groups or individuals who are potential rescuers or who are in need of rescuing. There are many useful applications for integrating this project into existing social media platforms. For example, in times of disaster, bots could continuously surf these social media sites and scrape data to be passed to the model. Once passed to the model, content from social media sites can be flagged as either signal or noise, and if signal, rescuer or rescuee. This model would perform best as a feature on a social media platform such as Facebook. We would recommend imbedding the tool in messaging or private groups to use signal data.